4 LECTURE: The Data Science Pipeline
Watch a video of this lecture.
4.1 Introduction
The basic issue is when you read a description of a data analysis, such as in an article or a technical report, for the most part, what you get is the report and nothing else. Of course, everyone knows that behind the scenes there’s a lot that went into this report and that’s what I call the data science pipeline.
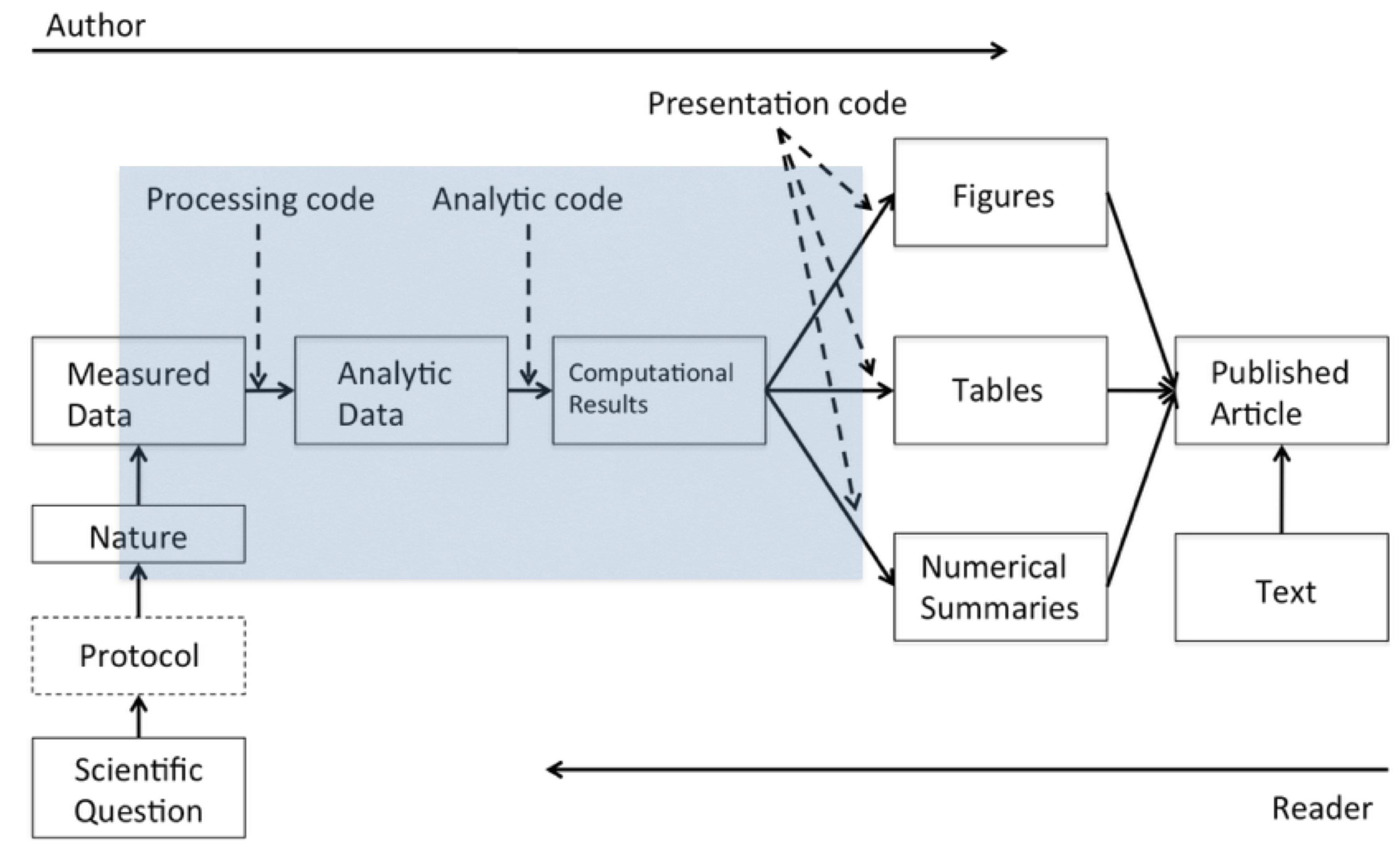
The Data Science Pipeline
In this pipeline, there are two “actors”: the author of the report/article and the reader. On the left side, the author is going from left to right along this pipeline. The reader is going from right to left. If you’re the reader you read the article and you want to know more about what happened: Where is the data? What was used here? The basic idea behind reproducibility is to focus on the elements in the blue blox: the analytic data and the computational results. With reproducibility the goal is to allow the author of a report and the reader of that report to “meet in the middle”.
4.2 Elements of Reproducibility
What do we need for reproducibility? There’s a variety of ways to talk about this, but one basic definition that we’ve come up with is that there are four things that are required to make results reproducible:
Analytic data. The data that were used for the analysis that was presented should be available for others to access. This is different from the raw data because very often in a data analysis the raw data are not all used for the analysis, but rather some subset is used. It may be interesting to see the raw data but impractical to actually have it. Analytic data is key to examining the data analysis.
Analytic code. The analytic code is the code that was applied to the analytic data to produce the key results. This may be preprocessing code, regression modeling code, or really any other code used to produce the results from the analytic data.
Documentation. Documentation of that code and the data is very important.
Distribution. Finally, there needs to be some standard means of distribution, so all this data in the code is easily accessible.
4.4 Summary
The process of conducting and disseminating research can be depicted as a “data science pipeline”
Readers and consumers of data science research are typically not privy to the details of the data science pipeline
One view of reproducibility is that it gives research consumers partial access to the raw pipeline elements.